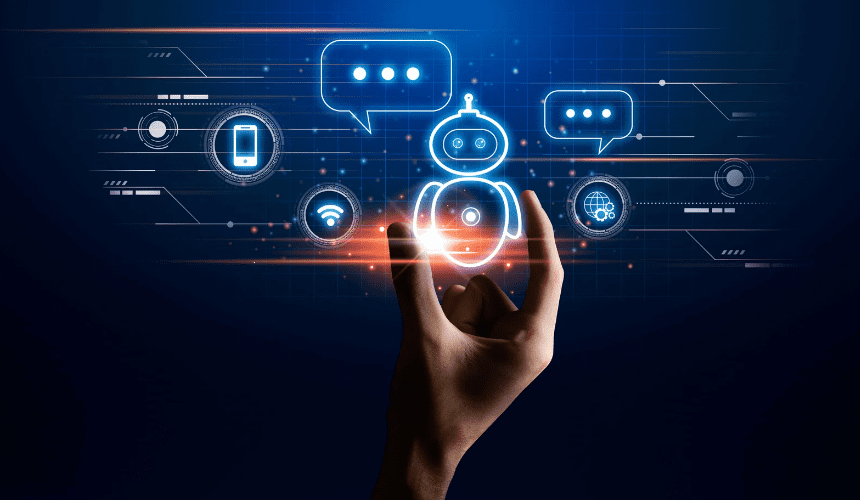
In the rapidly evolving field of artificial intelligence, the creation and refinement of prompts play a crucial role in shaping the performance and accuracy of AI systems. One of the most powerful tools in this process is the feedback loop, which enables continuous improvement through iterative adjustments.
By leveraging feedback loops, developers can fine-tune their systems to generate more precise and effective AI prompts, ultimately enhancing the overall performance of AI applications. This approach fosters a deeper understanding of AI capabilities and ensures that AI systems remain responsive and adaptable to changing needs and contexts.
In this article, we will explore how feedback loops contribute to the iterative improvement of AI performance, emphasizing the importance of crafting helpful AI prompts.
Understanding AI Prompts
At the core of AI interactions are prompts—the questions, commands, or inputs given to an AI model to elicit a response. The effectiveness of AI largely depends on how well these prompts are crafted. For instance, a vague or poorly structured prompt may result in inaccurate or irrelevant responses, while a well-designed prompt can yield precise and valuable outputs.
Prompts act as a bridge between human intention and machine understanding. They translate human queries into a form AI can process and respond to effectively. Therefore, the quality of prompts directly influences the AI’s ability to perform tasks such as natural language processing, image recognition, or decision-making.
The Role of Feedback Loops
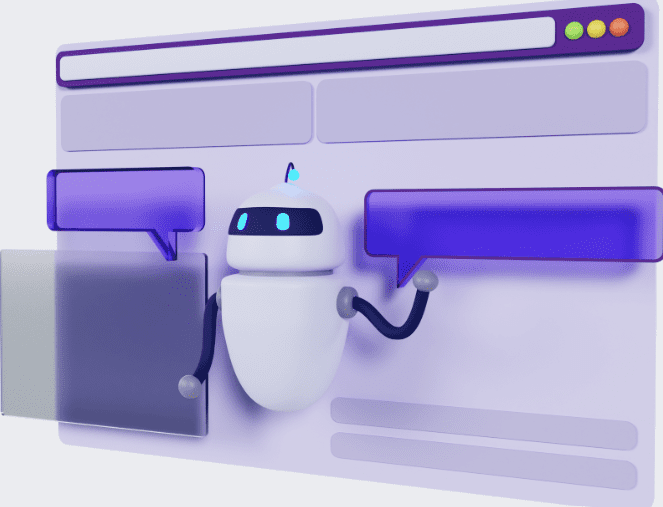
A feedback loop is a process where a system’s outputs are circled back as inputs, allowing continuous refinement and improvement. In the context of AI, feedback loops involve evaluating the responses generated by AI models, providing constructive feedback, and adjusting the prompts accordingly. This iterative process helps fine-tune the AI system, making it more accurate, reliable, and efficient.
Feedback loops are essential because they create continuous learning and adaptation cycles. By regularly analyzing AI responses and modifying prompts based on feedback, developers can address shortcomings and enhance the system’s performance.
Steps in an Effective Feedback Loop
- Initial Prompt Design: The first step is crafting a set of prompts based on the desired outcomes. This involves understanding the task’s context, objectives, and specific requirements.
- Response Evaluation: Once the AI generates prompt responses, these outputs are evaluated for accuracy, relevance, and quality. This evaluation can be done through manual review, automated systems, or both.
- Feedback Generation: Based on the evaluation, feedback is generated to highlight areas of improvement. This feedback can be specific, pointing out errors or suggesting modifications, or it can be more general, providing overall performance insights.
- Prompt Refinement: Using the feedback, the prompts are revised and improved. This might involve rephrasing questions, adding context, or breaking complex queries into simpler components.
- Reiteration: The refined prompts are tested again, and the cycle continues. Each iteration aims to bring the AI responses closer to the desired quality and accuracy.
Benefits of Feedback Loops in AI Prompt Refinement
1. Improved Accuracy and Relevance
Feedback loops help in honing prompts to be more precise and contextually relevant. As a result, AI systems can generate responses that are more aligned with user expectations and requirements. This accuracy is crucial in applications like customer support, where accurate and relevant responses are vital.
2. Enhanced Learning and Adaptability
Continuous feedback enables AI models to learn and adapt over time. With each iteration, the system becomes more adept at understanding the nuances of prompts and delivering better responses. This adaptability is essential in dynamic environments where requirements and contexts frequently change.
3. Reduced Bias and Errors
AI systems are susceptible to biases and errors, which can be mitigated through effective feedback loops. By consistently evaluating and refining prompts, biases can be identified and corrected, leading to more fair and unbiased AI outputs. Additionally, errors can be minimized, enhancing the system’s overall reliability.
4. Greater User Satisfaction
User satisfaction naturally increases when AI systems deliver accurate, relevant, and timely responses. Feedback loops are crucial in achieving this by ensuring that the AI consistently meets user expectations. Satisfied users are likely to trust and rely on AI solutions, driving higher adoption rates.
Practical Applications of Feedback Loops
1. Customer Support
In customer support, AI-driven chatbots and virtual assistants rely heavily on prompts to interact with users. Feedback loops enable these systems to improve their conversational abilities, providing more accurate and helpful responses. By continuously refining prompts based on user interactions, businesses can enhance customer support services, increasing customer satisfaction and loyalty.
2. Content Generation
AI models used for content generation, such as writing articles, creating marketing copy, or generating social media posts, benefit significantly from feedback loops. Writers and marketers can evaluate the AI-generated content, provide feedback, and refine prompts to ensure the output aligns with their brand voice and messaging. This iterative process leads to higher-quality content that resonates with the target audience.
3. Healthcare
AI diagnoses diseases, recommends treatments, and manages patient care in healthcare. Accurate prompts are crucial to ensure reliable AI outputs. Feedback loops allow healthcare professionals to continuously improve the prompts, leading to more accurate diagnoses, personalized treatment plans, and better patient outcomes.
4. Education
AI-powered educational tools like tutoring and personalized learning platforms rely on effective prompts to guide student interactions. Feedback loops help refine these prompts, ensuring the AI provides relevant and engaging educational content. This enhances the learning experience and improves student performance.
Conclusion
The iterative improvement of AI prompts through feedback loops is a powerful method to enhance AI performance. Developers can achieve higher accuracy, relevance, and reliability in AI outputs by continuously evaluating AI responses, providing feedback, and refining prompts. This process is crucial across various applications, from customer support and content generation to healthcare and education.
Feedback loops create a cycle of continuous learning and adaptation, allowing AI systems to evolve and meet user expectations effectively. As AI advances, the importance of well-crafted prompts and effective feedback loops will only grow, driving better AI performance and greater user satisfaction. Embracing this iterative approach is critical to unlocking AI’s full potential and harnessing its benefits across diverse industries.